Evolving Human-in-the-Loop: Building Trustworthy AI in an Autonomous Future
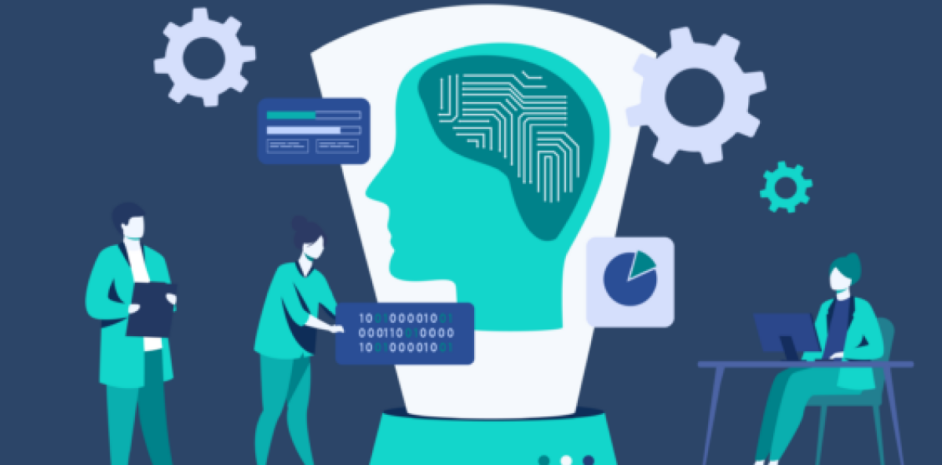
Key takeaways
- As AI systems evolve toward more agentic and autonomous workflows, the role of humans will inevitably change—but their oversight will remain critical.
- Human-in-the-loop (HITL) involves humans acting as teachers to AI models, instructing them on how to interpret data, make decisions, and respond appropriately in real-world applications.
- Moving from operational control to strategic oversight, agentic AI systems will incorporate human-in-the-loop interactions at key stages when human expertise or approval is required.
- This new dynamic lifts much of AI’s operational burden off humans, while maintaining the accuracy and expertise enterprises require.
- From healthcare and customer service to AI development itself, more autonomous AI systems with strategic HITL are enhancing productivity across industries and use cases.
Understanding human-in-the-loop
Human-in-the-loop is the process of integrating human input at various stages of an AI system’s lifecycle. This involves human experts correcting, guiding, and improving the AI model through feedback, much like a teacher guiding a student.
Stages of HITL in development:
Model training and re-training: Humans, often developers, teach AI models how to interpret data and make predictions. They correct AI outputs and adjust model behavior to improve alignment with use case requirements.
Pre-production testing: Before deployment, models undergo beta testing with human domain experts who refine the model further. Feedback from these experts fine-tunes the AI’s performance to prepare it for real-world implementation.
Post-deployment feedback: Once in production, humans continue to provide feedback on suboptimal responses. This feedback loop helps the AI model learn continuously and improve over time.
Human-in-the-loop helps enterprises build more intelligent AI
In enterprise AI, accuracy and transparency are non-negotiable. Human-in-the-loop helps meet these criteria in several ways:
Achieving accuracy: AI systems need continuous supervision to ensure outputs are correct. HITL allows human experts to detect errors and correct model behavior to decrease these instances in the future.
Building trust through transparency: Enterprises need AI systems that stakeholders—whether customers, employees, or regulators—can trust. Incorporating human oversight into the AI lifecycle helps enterprises understand and explain the decision-making process and foster transparency.
Where HITL gets complicated
While HITL is mandatory to build accurate enterprise applications, it does present operational challenges:
Finding domain experts: AI developers may be experts in their own field, but they can’t always train models to meet the accuracy requirements of specialized domains like legal or healthcare. Recruiting subject matter experts, such as doctors or lawyers, who can dedicate time to providing model feedback creates a bottleneck.
Human biases in training: Humans introduce their own biases when training models, which can lead to unfair decision-making and unethical responses. Involving a diverse set of humans helps balance biases and reach an acceptable average.
How to successfully integrate HITL into your workflow
Teams should understand and implement the latest HITL techniques to overcome hurdles and streamline model optimization with human feedback:
Reinforcement learning from human feedback (RLHF): This method uses a reward system to reinforce desirable behaviors and discourage undesirable ones in model outputs. Humans evaluate model responses based on criteria like helpfulness, appropriateness, and relevance to align the model closer with human values and expectations. This technique is particularly useful for conversational AI applications where the model will interact with humans in nuanced or open-ended ways.
Preference-based learning: In preference-based learning, humans compare two model outputs side-by-side and select the one they prefer. The model then starts to prioritize these types of outputs and refine its behavior based on these aggregate user preferences.
Active learning: In this HITL strategy, the model sends responses for human review when it identifies uncertainty or lacks confidence in its outputs. This method is effective because it makes the most efficient use of human resources.
Thoughtful UX design: Creating user experiences that make it easy for humans to provide feedback is essential to encourage more feedback and ensure it’s being collected and implemented effectively. This supports the continuous feedback loop that plays a key role in optimizing model performance in production.
How will human-in-the-loop change in an agentic future?
As AI evolves toward more autonomous systems, the relationship between humans and AI will change—but how?
In this agentic future, self-governing AI systems will take on many tasks currently managed by humans, including data creation, model training, and retraining. But this doesn’t eliminate the need for human oversight.
Learn more about the power of agentic AI
Read MoreStrategic oversight over operational control
Human involvement will become more focused on high-level guidance. AI agents will handle routine decision-making processes and call on humans to step in when they encounter more ambiguous or high-stakes scenarios. This selective approach allows AI to self-govern most of the time while keeping humans involved when it matters most.
Agents-in-the-loop
Teams will be able to train AI agents to behave like human domain experts and train other AI models based on their expertise—essentially creating an “agent-in-the-loop” workflow.
Imagine an agent that is trained in Shakespeare from the knowledge of a human literary expert. This agent could guide the training of another model focused on specific aspects of Shakespeare’s works, leveraging its expertise to produce a more refined outcome.
The workflow is: agents training agents based on human expertise.
Real-world examples of human-agent collaboration
Several industries are beginning to leverage new, advanced AI workflows to optimize solutions for efficiency and accuracy. In these applications, the AI handles tasks more autonomously while the human remains in the loop to review outputs, provide corrective feedback, and make final decisions.
1. Customer service automation with selective human oversight
In customer support, AI virtual agents are taking over routine queries to produce faster response times and reduced operational costs. However, human agents remain in the loop to handle more complex or sensitive interactions. For example, if an AI agent encounters a nuanced customer complaint that requires emotional sensitivity, the interaction is escalated to a human agent to take over.
2. Automated medical diagnostics with human clinician review
In healthcare, agentic AI systems can be used to analyze medical images, detect patterns that may be difficult for humans to spot, and generate diagnostic insights and personalized treatment plans. Human medical professionals then review the results to confirm their accuracy, provide model feedback if necessary, and make the final decision before sharing with the patient.
3. Agentic data creation with data scientists in the loop
In AI development, data preparation is arguably the most challenging and time-consuming step in the process. Now data scientists can automate data generation, synthesis, augmentation, labeling, and curation through an autonomous workflow in the SeekrFlow™ AI platform.
This agentic-based system allows teams to provide the AI agent with the high-level principles and company policies they want the model to adhere to. The agent will then autonomously generate a high-quality training dataset. Data scientists can then review this dataset, make corrections, and optimize it before beginning the fine-tuning process.
Human-in-the-loop will shape the next era of enterprise AI
Human-in-the-loop processes will remain a cornerstone in building trusted AI applications that are accurate, ethical, and aligned with business goals. As we move toward a more autonomous future, implementing strategic HITL techniques will provide the essential guidance needed to ensure safe, effective automation and foster more reliable AI solutions.